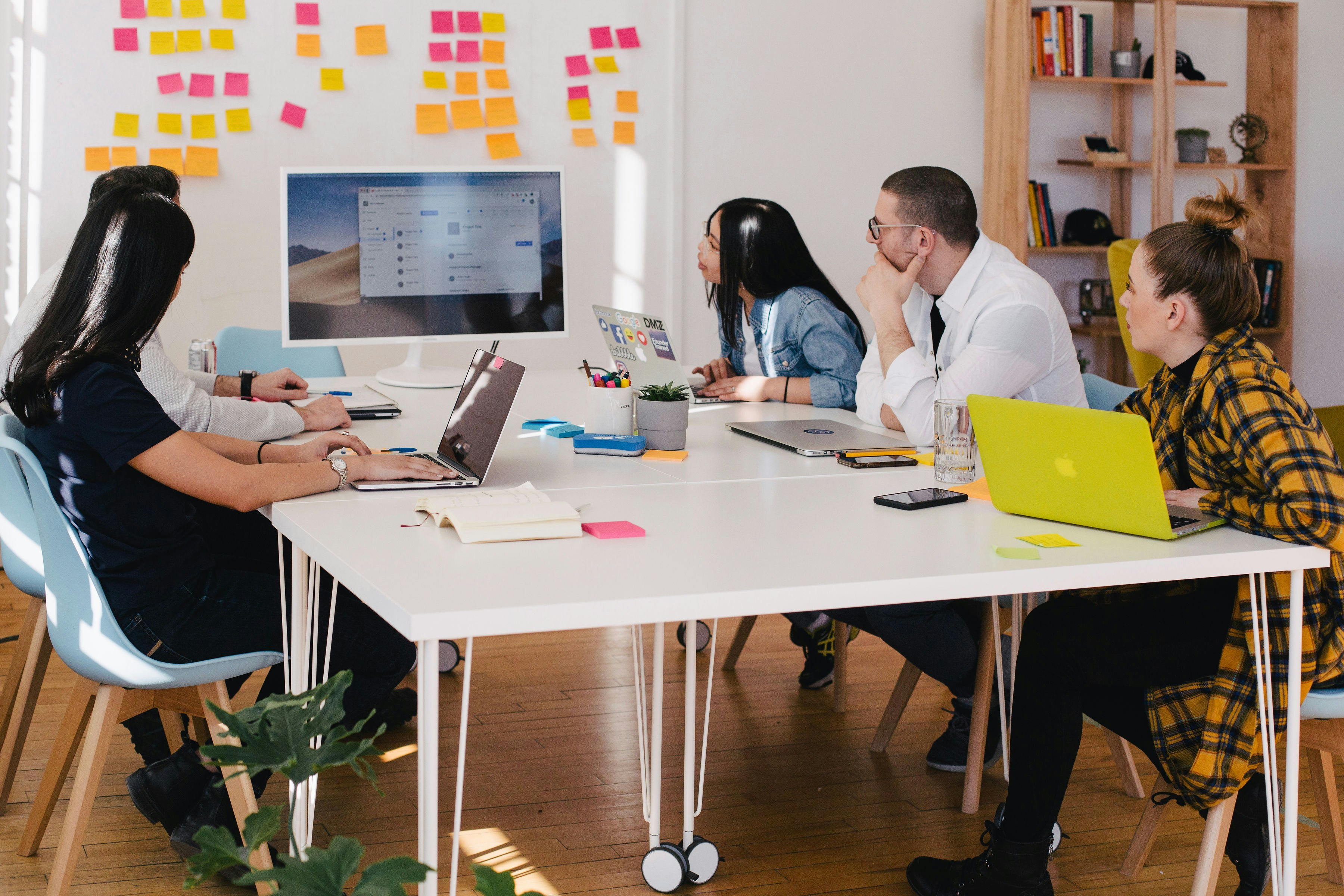
Because we specialize in helping partners from ideation to launch, we have a lot of practice with early-stage business ideas and navigating their growth to full-blown businesses. We’ve had a lot of recent inquiries about AI ideas, so we thought we’d outline the process of taking an idea through Product-Solution Fit, Product-Market Fit, and scaling to Business Model Fit. In this post, we’ll focus on Product-Solution Fit since it’s the first step.
Before getting into any one of these, it’s helpful to know what they each mean at a high level. The simple answer is that Product-Solution Fit means you can usefully solve a problem for a small handful of customers, whereas Product-Market Fit means that you’ve identified a large pool of customers for whom your solution works – your market. Finally, Business Model Fit means that you’re able to pull it all together into a working business that achieves profitability and growth. If Product-Solution Fit is fixing one person’s car, then Product-Market Fit is becoming a full-time mechanic, and Business Model Fit is becoming profitable enough to successfully open a second location.
For brand new ideas, we always want to start with Solution Fit. If we can’t get Solution Fit, we aren’t going to find Market Fit – at the end of the day, users want people who will solve a problem for them. Ideally, we want to prove or disprove Solution level fit quickly, so that we can either move forward in finding Product-Market fit or cut our losses before investing too much in a product that doesn’t have a future. Fast failing is no fun, but it certainly beats a slow, expensive failure. With this goal in mind, there are a few things that we always plan for when we’re looking for Solution Fit on a new product idea.
The core value
The first thing is to be crystal clear about the problem we’re solving, what value it creates for users, and what the solution will cost users. Cost can be financial, but it can also be time or inconvenience. For example, one of Google’s most notorious product failures, Google Plus, underestimated its cost to users – while the service was free, it also came with the inconvenience of having to maintain yet another social networking presence. Being clear-eyed about value versus full costs has three advantages. First, we can minimize secondary costs such as inconvenience as much as possible. Second, we can have realistic conversations about the value we’ll need to deliver to outweigh them. And third, we can use tools like User Research to quickly and efficiently test these sorts of tradeoffs with our target customers. This testing allows us to confirm our value, pivot, or realize that the value gap is simply too large and move on to the next thing.
Known unknowns
The next thing is to break our plan down into the individual things that have to work out in order to solve the problem for our initial users. For example, let’s say we’re building a tool to recommend products to teenagers. For this to work, we need:
- A way to discover age-appropriate products that we can recommend
- For each product, a way to get images and descriptions to make a visually compelling recommendation
- An app or webpage that teenagers can use to discover the products (but really an app, because teenagers are very mobile first)
- At least some teenagers to be predictable enough that good recommendations are possible
- An algorithm that can make good predictions for those teenagers
- A way to identify those predictable teenagers
Once we’ve broken the problem down into these concrete items, we can identify the best way to test each one. We can also estimate how much risk it poses to our overall plan.
Some requirements – for example, that an algorithm exists – are best tested by building engineering prototypes. Others – for example, that some teenagers have predictable preferences – can be cheaper to test via User Research in parallel with engineering prototype efforts. In each case, thinking about the most efficient way to test allows us to manage costs while quickly gaining information about our product space. In parallel with building out our test plan, we can estimate how much risk actually exists for that requirement. In this example, it’s a safe bet that we could build an app with product recommendations, but it’s potentially riskier to bet on teenage predictability.
By being intentional and quantitative about the cost and rough odds of each piece, we can front load cheaper and higher risk testing while leaving higher cost or high confidence steps for later in the process. We can also be thoughtful about how much we want to parallelize testing, in order to have a faster but potentially costlier project, versus run our tests one at a time in order to minimize costs.
Putting it all together
Once we’ve convinced ourselves that the core unknowns are good bets, the next step is to build a Minimum Viable Product (MVP) solution and prove that we can actually solve the problem at hand. If we’ve done a good job identifying all the major questions, then this step should be lower risk; we’ll have confidence that each piece works and the investment in a full MVP is merited. That said, there’s still a lot of elbow grease. Users need a solution that is intuitive and fully featured, and this step is where we make sure to deliver on that.
Next Steps
Hopefully, everything goes well and we find Product Solution Fit, which means the next step is Product Market Fit. That’s a subject for another blog!
Do you have a new business idea you’re kicking around and would like to test? Contact us and let us know what areas of product development interest you more for future blogs.